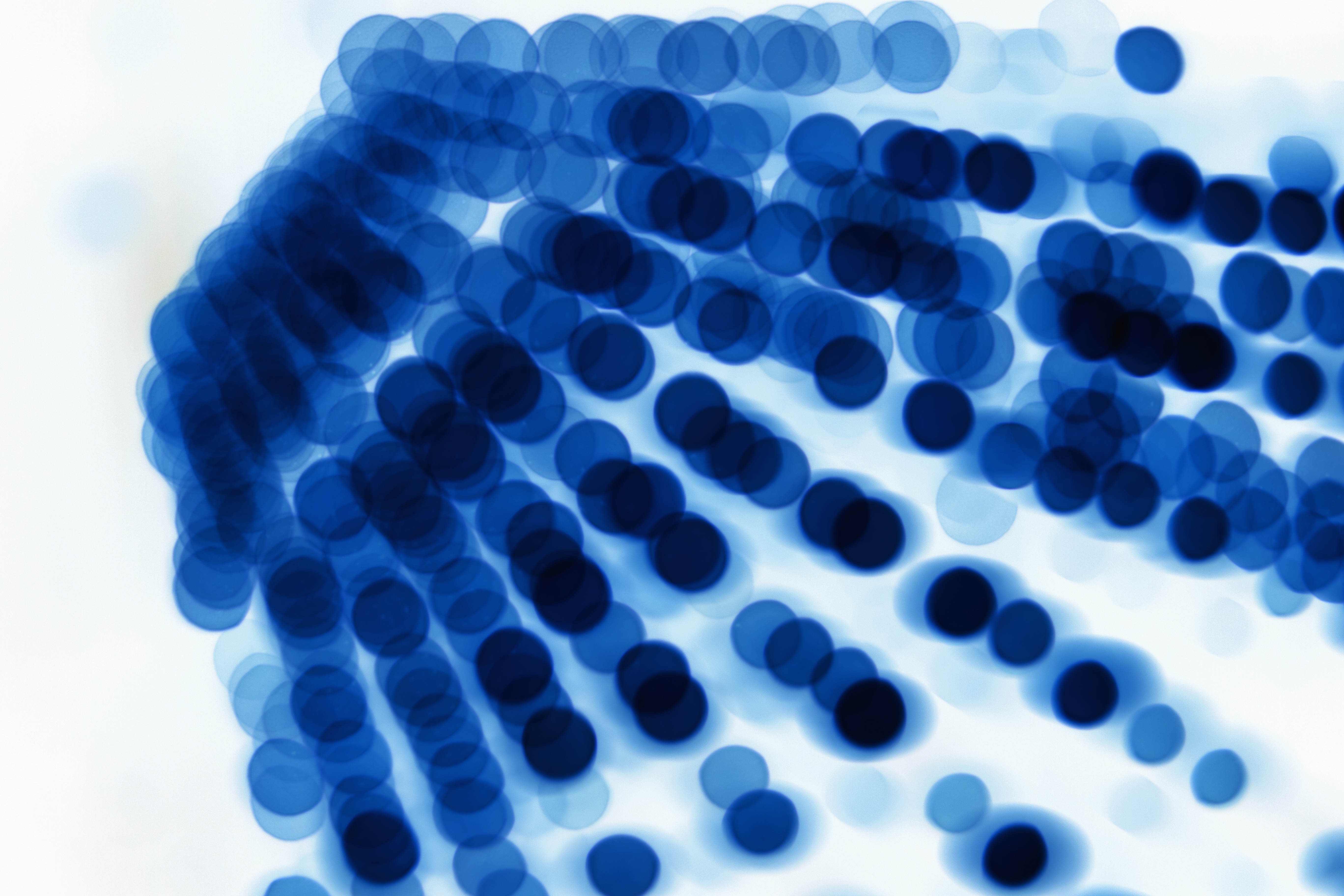
RNA sequencing (RNA-seq) is a powerful application of next-generation sequencing (NGS) technology that provides researchers with an alternative window into life’s central dogma. While DNA sequencing enables close interrogation of DNA-based variation and gene coding, it can only ever infer which of an organism’s genes are being expressed at any given time. In contrast, RNA-seq allows researchers to observe the temporal and spatial dynamics of gene expression, highlighting methods of gene regulation and, at times, shining invaluable light on mechanisms of disease.
Over the last decade, the evolution of NGS technology has led to a rapid and ongoing period of innovation, one in which novel applications for the technology are regularly being developed. RNA-seq is no exception. In recent years, expanding access to sequencing technology has enabled wider adoption and, accordingly, an increasingly diverse list of applications that span both clinical, industrial, and academic ventures. RNA-seq technology helps researchers identify public health threats, explore the fluid nature of cell identity, and call forth the chimeras that give rise to cancer, among many other such applications.
In this blog, we explore the current state of RNA-seq, giving a brief overview of the technology and the ways in which it is being applied, as well as tools that enable researchers to reap the benefits of this fast improving technology. To provide a comprehensive list of RNA-seq applications is a task worthy of a textbook. Instead, we have selected a few applications that demonstrate the range of RNA-seq’s benefits and, we believe, indicate where the technology is likely to become integral in the coming years.
What is RNA-seq
As described above, RNA-seq leverages NGS technology for hypothesis-free detection of RNA transcripts, enabling researchers to catalog the expression of mRNAs, micro-RNAs, long-noncoding RNAs, circular RNAs, and myriad other RNA species. Akin to DNA sequencing, the power of RNA-seq lies in its ability to generate vast troves of genetic data through relatively simple, high-throughput, and multiplexed workflows. Such data can then be used to profile cells, study the effects of genetic variation, and identify pathological processes.
Unlike DNA sequencing, RNA-seq allows researchers to study the dynamics of gene expression. Following transcription, RNA species may undergo a wide range of modifications—such as alternative splicing, transcript fusion, and transcript degradation—that significantly influences the final protein product, and none of which are easily predicted from DNA sequencing data. What’s more, the extent of these modifications is influenced by cellular context, such that a single cell in a dynamic environment may show considerably different transcriptomic profiles over time.
RNA-seq allows researchers to study these dynamic processes and begin to connect transcriptomic changes to cellular phenotypes. As we’ll discuss later, such studies can reveal the mechanisms by which cancer cells evade treatment; they can be used to trace the differentiation of stem cells over time; and they can even give rise to advanced diagnostics.
"Unlike DNA sequencing, RNA-seq allows researchers to study the dynamics of gene expression"
However, each of these applications requires a specialized set of tools to enable efficient and robust sequencing. RNA must be reliably retrieved from the sample, converted into cDNA, sequenced, and then reassembled and interpreted using bioinformatics. Challenges await researchers at every step. For example, patient samples may undergo formalin fixation prior to long term storage in paraffin wax, a process that makes it exceptionally difficult to retrieve sufficient amounts of viable RNA for sequencing. It is often necessary, too, to isolate specific RNA species from the complex milieu of transcripts that naturally exist within a cell, thus ensuring that sequencing resources are focused on only the desired and potentially rare transcripts. It is therefore important—for experimental efficiency, sensitivity, and robustness—that researchers have access to high-quality sequencing tools, such as library preparation kits, rRNA depletion kits, and target enrichment panels.
Twist Bioscience has developed an extensive suite of RNA-seq tools to support researchers across many different applications. You can explore these tools at this link: https://www.twistbioscience.com/products/ngs/rna-sequencing-solutions?tab=overview{{/a:1}}. As tools like those offered by Twist have developed, so too have the ways in which RNA-seq is being used.
Trending Applications of RNA-seq
From the evolution of malignant cells to the interrogation of wastewater, the list of research topics benefiting from RNA-seq seems to be increasing each year. Below are a select few, chosen to highlight the growing value of RNA-seq across diverse applications.
Detection of Environmental RNA for Public Health
Genetic material is continually shed from cells, pervading the body’s tissues and absconding into our environment. Relative to DNA, RNA is short lived outside of the body. RNAses in water and soil work fast on these single-stranded molecules, digesting them into short, unintelligible bits of nucleic acids1. However, when encased in a protective protein capsule, RNA can survive in the environment for extended periods of time. Such is the case when RNA viruses, laden with their unique genetic payload, are shed from infected organisms, expelled with a cough, sneeze, or, in some cases, the flush of a toilet. Thanks to RNA-seq, these wayward bits of viral RNA can be a helpful guide to public health efforts2-6.
During the COVID-19 pandemic, RNA-seq was increasingly adopted for wastewater based epidemiology, wherein samples of wastewater influent (collected from sewage treatment facilities or drainage) are used to monitor for viral species content, spread, and evolution at a population level2-5. Such an approach not only allows public health officials to detect the rise of viral infections and new variants before they’re observed in clinical settings, it also has the potential to identify asymptomatic viral spread. This information can then guide the distribution and enactment of preventative public health measures.
To do this, researchers may rely on myriad RNA-seq tools, including target capture panels that help to isolate RNA transcripts from complex solutions7,8. Twist has developed infectious disease panels such as the Comprehensive Viral Research Panel—built with over 1 million unique probes to capture 3,153 viral genomes—to enable the isolation and sequencing of viral genetic material. Twist has also developed safe, synthetic RNA controls to enable quality control of viral testing.
Note that similar applications are being developed to detect the spread of bacterial pathogens using next-generation sequencing for wastewater-based epidemiology9. And, beyond its application in public health, RNA-seq is fast becoming a valuable tool in the detection of macro- and microfauna in ecological settings based on environmental samples. You can read more about these applications here and here.
Tumor Detection Through Circulating Tumor RNA (ctRNA)
Naturally, RNA-seq has become a prolific tool in the study of cancer, often wielded to build high resolution profiles of tumor cell types and to interrogate the processes that so define them. However, as adoption of the technology has increased, so too have the ways in which it is used to study cancer. Beyond simply cataloging differences in gene expression, RNA-seq is now being used (1) to illuminate locoregional tumor heterogeneity (via spatial transcriptomics); (2) as a way to discover novel RNA-transcripts and their function in disease (thanks to long-read sequencing advances); and (3) as a potentially non-invasive diagnostic tool through the detection of circulating tumor RNA.
网络研讨会 | 基于组合的传染病测序策略
Learn how one research team is using panel-based sequencing to efficiently recover genomes from environmental samples and human samples, all to study infectious agents.
Cell-free nucleic acids are increasingly important sources of information in cancer research and diagnostics. Both DNA and RNA species can be found circulating through blood plasma and other bodily fluids, carrying with them valuable information about their cell of origin. For example, a patient’s blood plasma can be collected following curative anti-cancer treatment and analyzed for the presence of circulating tumor DNA (ctDNA)—identifiably by the presence of tumor-defining mutations—as an indirect measure of tumor cell persistence or recurrence (so called minimal residual disease testing)10. Relative to tumor biopsies and imaging techniques, assaying for cell-free nucleic acids is a far less invasive approach to patient testing and is amenable to long-term serial monitoring. Therefore several efforts are underway to develop diagnostic assays centered on ctDNA sequencing.
Similarly, capturing and sequencing extracellular RNA is proving to be a valuable tool for tumor detection and profiling, offering several potential advantages over ctDNA. Depending on the gene’s expression level, ctRNA transcripts may outnumber the corresponding ctDNA fragments, enabling deeper sequencing and more sensitive detection of diagnostic markers. Additionally, subtle changes in ctRNA expression levels can be used to assess tumor evolution over time and to detect developing therapeutic resistance11,12.
Of particular interest here is the detection of circulating microRNAs (miRNAs). Unlike many other forms of RNA, miRNAs are highly stable in the extracellular environment, due in large part to their association with protein complexes and exosomes (roughly 90% of circulating miRNAs are bound to proteins). The precise function of extracellular miRNAs is not fully understood, but it’s believed that they may act as both signaling molecules and tools for intercellular genetic regulation. For instance, miRNA-21 has been documented to be released by cancerous cells and to subsequently interact with distal cells, triggering in them a cascade of events that culminates in increased telomerase activity and chemoresistance 13.
"miRNA expression tends to be highly tissue and context specific"
Like other transcripts, miRNA expression tends to be highly tissue and context specific. Therefore the presence of a specific miRNA—or a collection of miRNAs—can be indicative of organ-specific disease. Accordingly, several miRNAs have already been linked to various tumor types and have demonstrated potential as early diagnostic biomarkers 11,14. (Note that the value of cell-free RNA as a diagnostic marker extends well beyond the context of cancer15.)
As a relatively new area of study, there is still much to be learned about cell-free RNA in health and disease. Continued investment in studying cell-free RNA species, along with increased access to RNA-seq tools, is likely to generate considerable discovery in this space over the next decade16.
Identifying Novel Transcripts and Disease Drivers
Technical limitations of current short-read sequencing workflows make it impractical for most labs to detect certain forms of genetic variation—in particular structural variants—at both the DNA and RNA levels. However, such variants pervade the human genetic landscape. In contrast, long-read sequencing technology is well suited to detecting structural variants and, thanks to recent advances, is seeing wider adoption for various applications, including the study of tumor transcriptomics.
Long-read RNA sequencing reduces the guess-work when mapping sequencing reads, enabling researchers to confidently reassemble phased, whole transcripts. As such, complex molecules—such as those containing aberrant splicing, duplicated or lost exons, and chimeras—can be reliably detected and quantified. Recent exploration of the tumor transcriptome using long-read sequencing has indeed revealed a complex and dynamic landscape of transcript variation, from splice variants to post-transcriptional and combinatorial gene fusion events, many of which are novel 12,17-20. For example, the recent use of long-read RNA-seq to survey the transcriptome in a collection of breast cancer cell lines (both immortalized and patient derived) resulted in the identification of 142,514 unique full-length transcript isoforms, approximately 80% of which were novel22.
That gene fusions can originate at the transcript level is a relatively new discovery, but one whose supporting evidence base is growing22,23. There is much to be learned about how such fusion events occur and their functional consequences. Fortunately, with greater access to long-read RNA-seq, the next decade should see a substantial expansion in our understanding of human transcriptomic variation.
Bringing Tissue & Cellular Heterogeneity To Light
The past decade has brought an explosive growth in multiomics technology with a general trend towards increasing resolution, throughput, and measured biological modalities. As alluded to in previous sections, DNA sequencing and RNA-seq are individually powerful research tools, however they are also narrowly focused on information coded in their respective biological materials and are often done on bulk-cell populations. Such a narrow focus ignores the inherently linked and interconnected nature of DNA, RNA, protein, and the cellular microenvironment, and how the collaboration of these factors can lead to inter-cellular heterogeneity. Therefore a significant effort has been made in recent years to collect single-cell, multi-modal data with the intention of correlating patterns across the spectrum of life’s central dogma.
Towards this end, single-cell RNA-seq has proven to be a foundational technology, often forming the basis of multiomic projects. Through microfluidic or plate-based methods, individual cells can be isolated and subject to RNA-seq. This provides information on gene expression patterns among individual cells, enabling scientists to build detailed inferences about each cell’s proliferative status, activation state, and metabolic activity. Such information can be valuable when detailing the extent of tumor heterogeneity, annotating cellular differentiation and maturation processes, or when studying the impact of gene perturbation in large-scale CRISPR-screens.
Single-cell RNA-seq is also proving invaluable as a quality control tool when working with cells derived from human induced pluripotent stem cells (iPSCs). Human iPSCs represent a promising source of mature human cell lines for in vitro disease modeling and drug development, however the differentiation of iPSCs is often a stochastic process that produces a highly heterogeneous population of cells. In a recent analysis from Fernandes et al., single-cell RNA-seq revealed heterogeneity among a seemingly homogenous group of dopaminergic neurons that were derived using a common protocol for iPSC differentiation (rooted in directed differentiation). Though many of the cells possessed dopaminergic markers, transcriptomic analyses showed that there were four different distinct neuronal subtypes and two neuronal progenitor populations24. Such heterogeneity can lead to biological noise and erode reproducibility of subsequent studies25. This example demonstrates how single-cell RNA-seq can be an invaluable tool, not just for characterizing iPSC differentiation methods, but in the broader use of casting intercellular heterogeneity into sharper relief.
Building on the strength of single-cell RNA-seq, many researchers have developed multiomics platforms that seek to correlate transcriptomic profiles with patterns in genomic, epigenomic, proteomic, and spatial context data. We reference readers to several recent comprehensive reviews for information on specific multiomic technologies26,27.
网络研讨会 | 通过直接捕获向导 RNA 和靶向测序实现可扩展和组合的单细胞 CRISPR 筛选
Learn how researchers are using single-cell CRISPR screening strategies to study functional genomics.
Applications for multiomics technology are manifold, with the majority sharing the same underlying rationale: Through the analysis of distinct and complimentary data sets, multiomics studies enable a more granular organization of cell types and the high-resolution observation of cellular processes.
For example, multiomics data sets are facilitating the tracing of cellular lineage trees and the identification of clinically relevant subpopulations. In a 2019 study, for instance, combined transcriptomic, genomic, and methylome sequencing was used to identify a defined subpopulation of chronic lymphocytic leukemia cells whose methylation and transcriptomic profiles predicted therapeutic sensitivity28. Other applications include the cataloging of organ-specific cell types at different developmental stages, profiling of tumor heterogeneity with spatial resolution—enabling correlation of environmental factors with malignant phenotypes—and the methodical annotation of genomic elements (among many other applications)27.
The ongoing development of multiomics technologies and the growing list of public multiomics datasets suggests that the next decade will likely see ever more adoption of this technology across the molecular sciences.
Expanding Our Definition of A Protein Coding Gene
Following publication of the draft human genome in 2003, many researchers were surprised to find that the human genome appeared to only encode between 20,000 and 25,000 proteins. In these analyses, some basic assumptions were made about what constituted a relevant protein coding gene. Namely, genes were defined as sequences >300 nucleotides (>100 amino acids), that were flanked by start and stop codons and were non-overlapping with other open reading frames (ORFs)29-31.
Now more than two decades later, we know that these stringent definitions may have obscured a large number of coding sequences, ones whose products are only just now coming to light.
Technologies like RNA-seq and ribo-seq have shed light on what’s generally referred to as the “dark proteome.”This enigmatic class of proteins (so called microproteins) are translated from segments of RNA that were previously thought to be non-coding, such as long-non-coding RNA (lncRNA) and 3’ or 5’ untranslated regions (UTRs)29,31.
Briefly, ribo-seq is a form of RNA-seq wherein protein-bound RNA transcripts are isolated and subject to RNAse digestion. Following RNAse treatment, all that remains in solution is the RNA that had previously been bound by—and thus protected—by a protein. Because ribosomes are known to envelop RNA-transcripts, protecting ~29 nucleotides, it can be reasoned that fragments of RNA in this size range were likely sites where ribosomes were bound. This data can thus be used to identify which transcripts are interacting with ribosomes and are likely undergoing translation29,30.
"Technologies like RNA-seq and ribo-seq have shed light on the ‘dark proteome’"
Over the past decade, ribo-seq has been increasingly used to uncover novel protein coding sequences among supposedly non-coding transcripts31,32. And, at least some of these proteins appear to be functionally involved in determining cell behavior and phenotypes. Studies in fruit flies have found multiple microproteins (<32aa long) that participate in physiological development33. In mammalian cells, an increasing repertoire of microproteins have been reported to have significant roles in DNA repair, mitochondrial function, and RNA regulation (among others)34-37.
Whether a microprotein is functionally active or not, it may still exert an important influence on a cell. Cells will need to expend resources digesting unused peptides. As with regular proteins, microproteins may be variant and form pathological aggregates or else become unique antigens. There is much we don’t know about microproteins, but the past few years have witnessed a growing appreciation for these overlooked entities and their potential value in cell biology, disease pathology, and drug development. RNA-seq will undoubtedly continue to be a foundational technology in the study and discovery of microproteins.
The Tip of The Iceberg
As alluded to in the above sections, RNA-seq is being used for a wide range of purposes, from public health to novel protein discovery. To be sure, we have only scratched the surface with this blog and have necessarily omitted applications of RNA-seq that are no less important or exciting. However, throughout this blog we have referenced several in-depth reviews and articles, most of which can serve as strong entry points to these topics and more. And, if you’re interested to learn more about RNA-seq tools offered by Twist, you can do so here.
参考文献
- Parra-Arroyo, Lizeth, et al. “Degradation of Viral RNA in Wastewater Complex Matrix Models and Other Standards for Wastewater-Based Epidemiology: A Review.”TrAC. Trends in Analytical Chemistry, vol. 158, Elsevier BV, Jan. 2023, pp. 116890–90, https://doi.org/10.1016/j.trac.2022.116890.
- Rothman, Jason, et al. “RNA Viromics of Southern California Wastewater and Detection of SARS-CoV-2 Single-Nucleotide Variants.”Applied and Environmental Microbiology, vol. 87, no. 23, 22 Sept. 2021, www.ncbi.nlm.nih.gov/pmc/articles/PMC8579973/, https://doi.org/10.1128/aem.01448-21.
- Gupta, Pooja, et al. “Wastewater Genomic Surveillance Captures Early Detection of Omicron in Utah.”Microbiology Spectrum, vol. 11, no. 3, 15 June 2023, https://doi.org/10.1128/spectrum.00391-23.
- Stockdale, Stephen R., et al. “RNA-Seq of Untreated Wastewater to Assess COVID-19 and Emerging and Endemic Viruses for Public Health Surveillance.”The Lancet Regional Health - Southeast Asia, vol. 14, 1 July 2023, p. 100205, www.sciencedirect.com/science/article/pii/S2772368223000653?via%3Dihub, {{term_url:1}}
- Amman, Fabian, et al. “Viral Variant-Resolved Wastewater Surveillance of SARS-CoV-2 at National Scale.”Nature Biotechnology, vol. 40, no. 12, 18 July 2022, pp. 1814–1822, www.nature.com/articles/s41587-022-01387-y, https://doi.org/10.1038/s41587-022-01387-y.
- Mao, Kang, et al. “The Potential of Wastewater-Based Epidemiology as Surveillance and Early Warning of Infectious Disease Outbreaks.”Current Opinion in Environmental Science & Health, vol. 17, Oct. 2020, pp. 1–7, https://doi.org/10.1016/j.coesh.2020.04.006.
- “Clinical Metagenomics for Improved Surveillance and Diagnosis of Infections in New South Wales, Australia | Twist Bioscience.”Www.twistbioscience.com, www.twistbioscience.com/resources/webinar/clinical-metagenomics-improved-surveillance-and-diagnosis-infections-new-south.
- “Panel-Based Sequencing Strategies for Infectious Diseases | Twist Bioscience.”Www.twistbioscience.com, www.twistbioscience.com/resources/webinar/panel-based-sequencing-strategies-infectious-diseases.
- Kumar Siddharth Singh, et al. “Indian Sewage Microbiome Has Unique Community Characteristics and Potential for Population-Level Disease Predictions.”Science of the Total Environment, vol. 858, Elsevier BV, Feb. 2023, pp. 160178–78, https://doi.org/10.1016/j.scitotenv.2022.160178.
- Stejskal, Pavel, et al. “Circulating Tumor Nucleic Acids: Biology, Release Mechanisms, and Clinical Relevance.”Molecular Cancer, vol. 22, no. 1, Jan. 2023, https://doi.org/10.1186/s12943-022-01710-w.
- Byron, Sara A., et al. “Translating RNA Sequencing into Clinical Diagnostics: Opportunities and Challenges.”Nature Reviews Genetics, vol. 17, no. 5, Mar. 2016, pp. 257–71, https://doi.org/10.1038/nrg.2016.10.
- Breeshey Roskams-Hieter, et al. “Plasma Cell-Free RNA Profiling Distinguishes Cancers from Pre-Malignant Conditions in Solid and Hematologic Malignancies.”Npj Precision Oncology, vol. 6, no. 1, Nature Portfolio, Apr. 2022, https://doi.org/10.1038/s41698-022-00270-y.
- Ho, Phuong T. B., et al. “MicroRNA-Based Diagnosis and Therapy.”International Journal of Molecular Sciences, vol. 23, no. 13, June 2022, p. 7167, https://doi.org/10.3390/ijms23137167.
- Cisterna-García, Alejandro, et al. “Cell-Free RNA Signatures Predict Alzheimer’s Disease.”IScience, vol. 26, no. 12, Cell Press, Dec. 2023, pp. 108534–34, https://doi.org/10.1016/j.isci.2023.108534.
- Ainsztein, Alexandra M., et al. “The NIH Extracellular RNA Communication Consortium.”Journal of Extracellular Vesicles, vol. 4, no. 1, Taylor & Francis, Aug. 2015, pp. 27493–93, https://doi.org/10.3402/jev.v4.27493.
- Sun, Qiang, et al. “Long-Read Sequencing Reveals the Landscape of Aberrant Alternative Splicing and Novel Therapeutic Target in Colorectal Cancer.”Genome Medicine, vol. 15, no. 1, BioMed Central, Sept. 2023, https://doi.org/10.1186/s13073-023-01226-y.
- Cavelier, Lucia, et al. “Clonal Distribution of BCR-ABL1 Mutations and Splice Isoforms by Single-Molecule Long-Read RNA Sequencing.”BMC Cancer, vol. 15, no. 1, Feb. 2015, https://doi.org/10.1186/s12885-015-1046-y.
- Nattestad, Maria, et al. “Complex Rearrangements and Oncogene Amplifications Revealed by Long-Read DNA and RNA Sequencing of a Breast Cancer Cell Line.”Genome Research, vol. 28, no. 8, Aug. 2018, pp. 1126–35, https://doi.org/10.1101/gr.231100.117.
- “Long-Read Sequencing Reveals More of the Tumour Transcriptome.”Www.nature.com, www.nature.com/articles/d42473-022-00248-1.
- Diogo F.T. Veiga, et al. A Comprehensive Long-Read Isoform Analysis Platform and Sequencing Resource for Breast Cancer. no. 3, Jan. 2022, https://doi.org/10.1126/sciadv.abg6711.
- Barresi, Vincenza, et al. “Fusion Transcripts of Adjacent Genes: New Insights into the World of Human Complex Transcripts in Cancer.”International Journal of Molecular Sciences, vol. 20, no. 21, Oct. 2019, p. 5252, https://doi.org/10.3390/ijms20215252.
- Dorney, Ryley, et al. Recent Advances in Cancer Fusion Transcript Detection. no. 1, Dec. 2022, https://doi.org/10.1093/bib/bbac519.
- Fernandes, Hugo J.R., et al. “Single-Cell Transcriptomics of Parkinson’s Disease Human in Vitro Models Reveals Dopamine Neuron-Specific Stress Responses.”Cell Reports, vol. 33, no. 2, Oct. 2020, p. 108263, https://doi.org/10.1016/j.celrep.2020.108263.
- Volpato, Viola, et al. “Reproducibility of Molecular Phenotypes after Long-Term Differentiation to Human IPSC-Derived Neurons: A Multi-Site Omics Study.”Stem Cell Reports, vol. 11, no. 4, Oct. 2018, pp. 897–911, https://doi.org/10.1016/j.stemcr.2018.08.013.
- Kashima, Yukie, et al. “Single-Cell Sequencing Techniques from Individual to Multiomics Analyses.”Experimental & Molecular Medicine, vol. 52, no. 9, Sept. 2020, pp. 1419–27, https://doi.org/10.1038/s12276-020-00499-2.
- Vandereyken, Katy, et al. “Methods and Applications for Single-Cell and Spatial Multi-Omics.”Nature Reviews Genetics, Mar. 2023, pp. 1–22, https://doi.org/10.1038/s41576-023-00580-2.
- Alev Baysoy, et al. “The Technological Landscape and Applications of Single-Cell Multi-Omics.”Nature Reviews Molecular Cell Biology, Nature Portfolio, June 2023, https://doi.org/10.1038/s41580-023-00615-w.
- Gaiti, Federico, et al. “Epigenetic Evolution and Lineage Histories of Chronic Lymphocytic Leukaemia.”Nature, vol. 569, no. 7757, 5月 2019, pp. 576–80, https://doi.org/10.1038/s41586-019-1198-z.
- Posner, Zoe, et al. “Shining a Light on the Dark Proteome: Non-Canonical Open Reading Frames and Their Encoded Miniproteins as a New Frontier in Cancer Biology.”Protein Science: A Publication of the Protein Society, vol. 32, no. 8, Aug. 2023, p. e4708, https://doi.org/10.1002/pro.4708.
- Ingolia, Nicholas T., et al. “Ribosome Profiling Reveals Pervasive Translation outside of Annotated Protein-Coding Genes.”Cell Reports, vol. 8, no. 5, Sept. 2014, pp. 1365–79, https://doi.org/10.1016/j.celrep.2014.07.045.
- Wright, Bradley W., et al. “The Dark Proteome: Translation from Noncanonical Open Reading Frames.”Trends in Cell Biology, vol. 32, no. 3, Mar. 2022, pp. 243–58, https://doi.org/10.1016/j.tcb.2021.10.010.
- Hassel, Keira R., et al. “Microproteins: Overlooked Regulators of Physiology and Disease.”IScience, vol. 26, no. 6, Cell Press, June 2023, pp. 106781–81, https://doi.org/10.1016/j.isci.2023.106781.
- Kondo, Takefumi, et al. “Small Peptide Regulators of Actin-Based Cell Morphogenesis Encoded by a Polycistronic MRNA.”Nature Cell Biology, vol. 9, no. 6, 7 5月 2007, pp. 660–665, https://doi.org/10.1038/ncb1595.
- Zhang, Qiao, et al. “The Microprotein Minion Controls Cell Fusion and Muscle Formation.”Nature Communications, vol. 8, no. 1, June 2017, https://doi.org/10.1038/ncomms15664.
- D’Lima, Nadia G., et al. “A Human Microprotein That Interacts with the MRNA Decapping Complex.”Nature Chemical Biology, vol. 13, no. 2, Nature Portfolio, Feb. 2017, pp. 174–80, https://doi.org/10.1038/nchembio.2249.
- Rathore, Annie, et al. “MIEF1 Microprotein Regulates Mitochondrial Translation.”Biochemistry, vol. 57, no. 38, American Chemical Society, Sept. 2018, pp. 5564–75, https://doi.org/10.1021/acs.biochem.8b00726.
- Arnoult, Nausica, et al. “Regulation of DNA Repair Pathway Choice in S and G2 Phases by the NHEJ Inhibitor CYREN.”Nature, vol. 549, no. 7673, Sept. 2017, pp. 548–52, https://doi.org/10.1038/nature24023.
您怎么认为?
喜欢
不喜欢
喜爱
惊讶
有兴趣