Exome Sequencing 101: Part 4 – Data Analysis
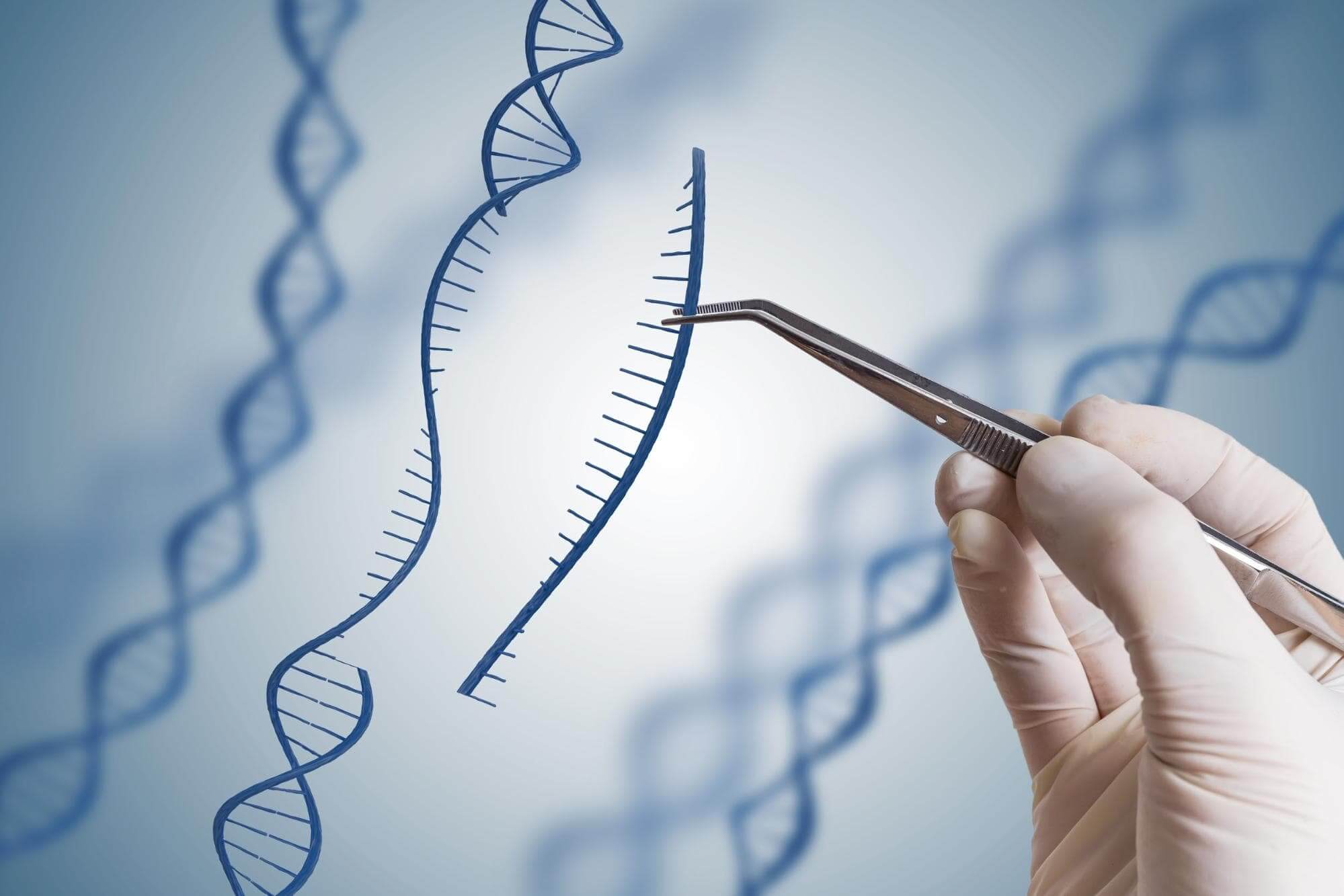
We have reached the final installment of our four-part series on next generation sequencing (NGS). In the previous three installments, we described the preparation of a genomic library, followed with an explanation of target enrichment procedures that isolate just the 1% of the genome that encodes the exome, and walked through the sequencing of the genomic sample, leaving us with a digital data file. In this piece, we’ll describe the last step in a typical NGS pipeline: making sense of the sequenced data.
Bioinformatics
We now return to the example we visited in the earlier installments of our NGS series. When we left off, clinical technicians had enriched the exome from a patient’s genome in order to investigate the cause of a mysterious disease by focusing their efforts on the 1% of the genome that codes proteins.
Now, in order to inspect the sequenced exome, the technicians have to apply a series of analysis methods to their data that will help them determine whether the patient’s genome includes a specific disease-causing mutation. These methods come from the field of bioinformatics. One large focus of bioinformatics is genomics - the handling of data that describes the genome, gleaned from a vast array of DNA sequencing experiments.
In the context of a NGS workflow, preparing the genomic library, enriching the target regions, and sequencing the DNA are all precursors to the bioinformatics grand finale, where data leads to insights, and research questions yield to answers.
The first step in the bioinformatics workflow involves cleaning the data and aligning the sequences to a reference genome. Often times, it is necessary to trim a number of nucleotide reads off of the ends of sequences, because these end regions are more vulnerable to misreading than the interior regions.
Once the data has been cleaned, the sequences must be aligned to a reference genome. Such a genome does not represent any single individual, but instead is compiled from many donors, so that variation in the sequenced data can be detected against a default genomic backdrop.
With the data cleaned and referenced, the sequencing data is ready for the main phase of data analysis. There are three main analysis targets a bioinformatician will likely focus on in their Exome sequencing workflow: sequencing quality, capture efficiency, and variance detection.
Variance Detection
For many NGS workflows, variance detection is one of the most important metrics, as it tells you the difference between your reference genome, and your sequenced genetic material. For example, in the context of exome sequencing and our patient example, the researchers could be trying to confirm the presence of a particular single nucleotide polymorphism, or sequence deletion event that is an indicator of early-onset Alzheimer’s disease. This type of mutation can be detected as a variant from the expected nucleotide sequence for the gene in question.
But before looking for variants, sequencing quality metrics and capture efficiency metrics must first be examined, so that the researcher can be confident in their assessment of the variance detection analysis. The researcher needs to know that they have the ability to capture the variance of interest, at the coverage depth they want to achieve.
Sequencing Quality
There are multiple important measures of sequence quality that can be gleaned from the FastQ file outputted from a high throughput sequencing experiment. Embedded in the FastQ file is data about the quality of the sequencing data itself, that report the probability of a base being read correctly by the sequencer.
Another important quality metric is read depth: the number of sequenced fragments that map to a given nucleotide. The industry standard for read depth in hybridization capture varies by the type of experiment being performed. For exome sequencing experiments, the coverage standard for confidence in an experiment is 20x – that is, 20 sequenced fragments align with a nucleotide of interest. This level of read depth increases the likelihood that the variants that were detected in a sequenced sample are true positive calls, and not false positives. But the industry standard for read depth can vary dramatically by the type of experiment. In searches for certain rare mutations, the industry standard can range as high as 2000x.
In an exome sequencing experiment, common metrics of importance include uniformity, off target capture events, and duplicate rate.
Uniformity allows a researcher to visualize how much of the captured exome has under, or over sequenced. Off target capture events are defined as sequences in the genome that were never meant to be captured, and inform a researcher about quality of capture library design. Duplicate rate assess how often certain fragments are captured more than once. If large percentages of certain targets are duplicates, it is possible to undersequence or even miss diversity from other targets.
Tools of the Trade
Several software platforms provide researchers with tools to assess the quality of their sequencing data, and detect variance of interest.
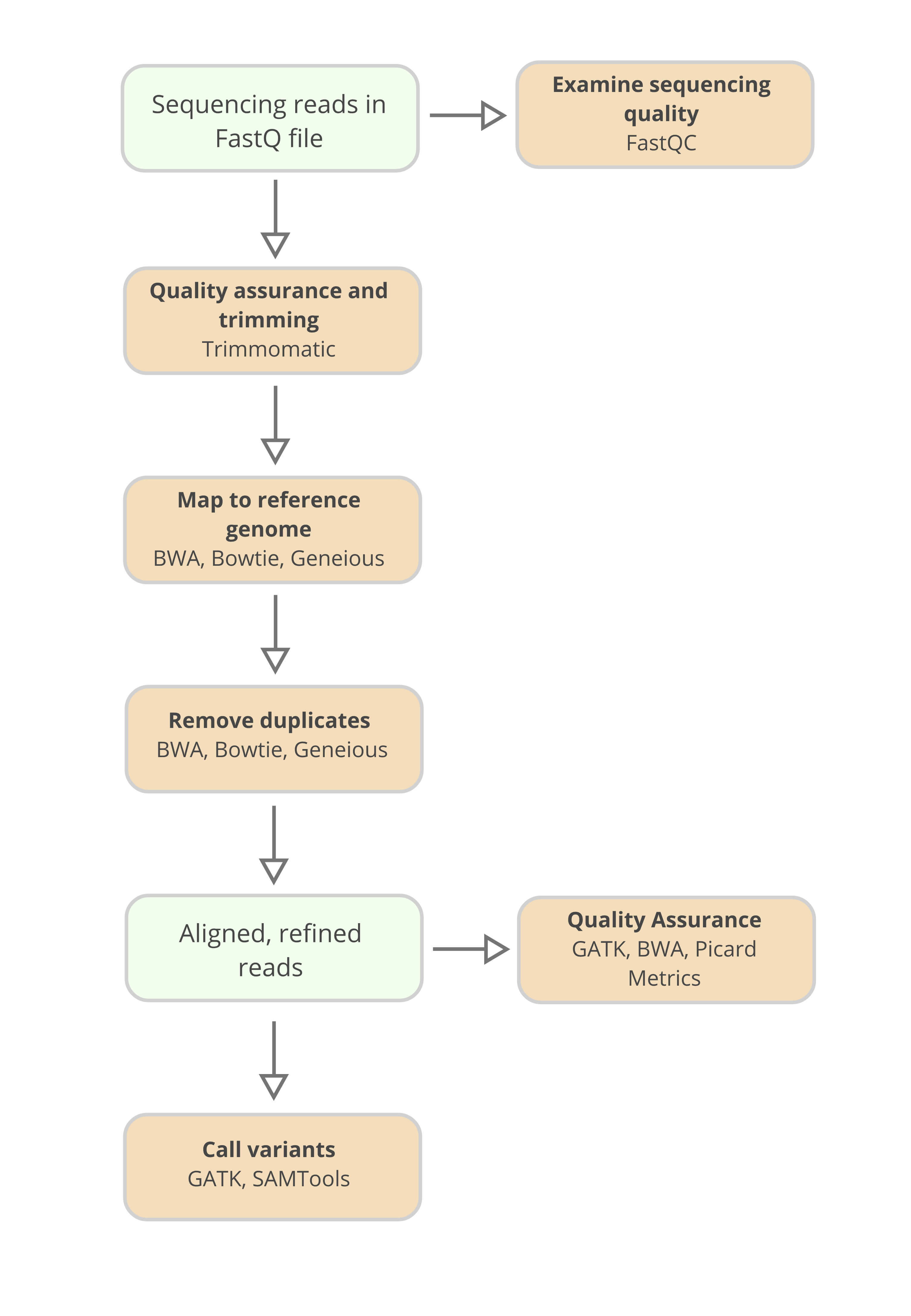
In order to easily perform quality control assessments on the data within the FastQ file, tools like FastQC are available. Poor quality data is then often cleaned from the FastQ file. Again tools exist to perform this function, for example Trimmomatic.
Numerous software tools, including Bowtie, the Burrows-Wheeler Aligner (BWA), and Geneious, allow researchers to align sequenced DNA to the portions of the reference genome from which they originated.
The Integrated Genome Visualization (IGV) tool, from the Broad Institute, is a popular choice for visualizing alignment data. The UCSC genome browser is another popular visualization tool for sequence databases. Both the IGV and the UCSC genome browser provide the same utility, and choosing between the two is often a matter of personal preference.
For quality assurance, open source software packages such as the Genome Analysis Toolkit (GATK), and Burrows-Wheeler Aligner (BWA) allow researchers to assess metrics from their sequencing experiment and clean sequences where necessary.
A common toolkit for assessing data quality is Picard Alignment Summary metrics. From this toolkit, detailed summary reports describing the quality of a sequencing experiment can be produced.
To call genomic variants, again, tools like GATK and SAMtools will assess the alignment and its read depth, and call single nucleotide polymorphisms, or indels where they occur in the sequenced sample.
Using these software platforms, the researcher can verify that sequencing quality meets the standards for the type of experiment being run, and that capture performance is sufficient. These benchmarks provide the solid foundation that is necessary to assess whether variants are present in the sequenced data.
But sometimes mistakes happen in the NGS workflow that can lead to bad read depth or inefficient capture performance. For instance, context-specific biases and non-uniformity can impact capture quality, especially in highly repetitive, GC-rich and non-unique regions of the genome. Or, probes could be designed inefficiently, diminishing on-target rate, sequencing depth, and overall capture uniformity.
Twist Bioscience’s target capture kits are a remedy to these problems. Twist’s core exome capture panel is designed to target 33 Megabases of genome based on the Consensus CDS project of high quality annotated genes. To optimize for uniformity, Twist’s probes are designed to account for capture efficiency. Synthesis and amplification are optimized at every stage, to control representation and ensure that both strands of the target sequence can be captured.
Now we return to the patient example that we’ve followed throughout this series. Luckily, the clinical technicians investigating the patient’s illness used Twist capture probes in their workflow, so their sequence quality assessment measures all indicate that their data is sound. Upon this strong foundation, the researchers were able to find a single nucleotide polymorphism associated with a disease mutation that fit their patient’s symptoms. Because of the speed and efficacy of their NGS workflow, they were able to prescribe medicine to the patient early enough in their disease to significantly improve the patient’s prognosis.
Here is the entire series:
Exome Sequencing 101: Part 1 – Library Preparation
Exome Sequencing 101: Part 2 – Target Enrichment
Exome Sequencing 101: Part 3 – Next-Generation Sequencing (NGS)
いかがでしたか?
気に入った
気に入らなかった
大好き
驚かされた
面白かった