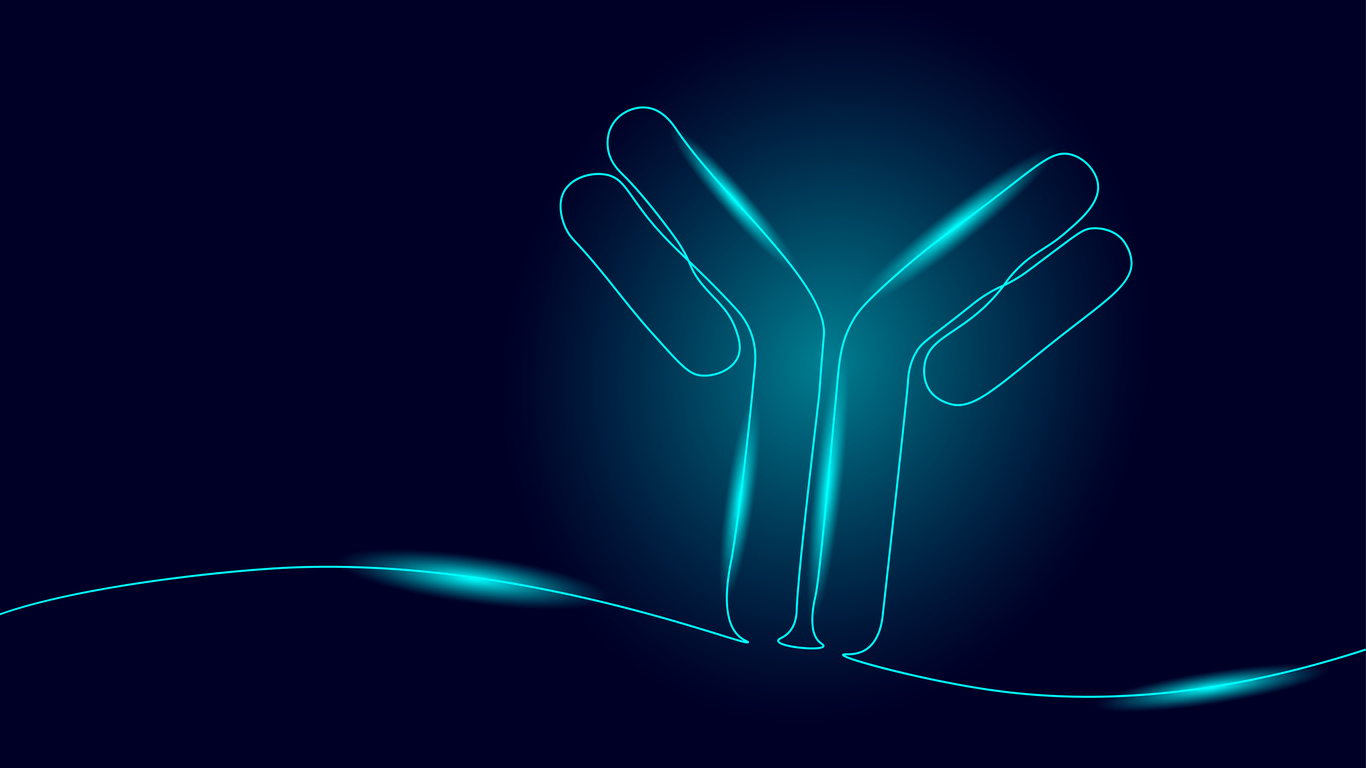
When beginning the search for therapeutic antibody candidates, researchers must often choose between discovery tools. Some opt to go with in vivo technologies like hybridoma and single-B cell sequencing that tap into natural antibody reservoirs, the depths of which have been molded by millions of years of evolution. Others turn to the in vitro powerhouse of display-based technologies or, increasingly, to powerful in silico modeling tools.
Often, researchers feel they have to choose between in vivo, in vitro, and in silico methodologies to find the solution that is best suited to their project. This can be a hard choice to make, especially when the workflow could benefit from their synergistic strengths.
To address this issue and to provide a superior drug discovery engine to the industry, Twist Biopharma Solutions recently launched a combined offering for antibody discovery, one that leverages in vivo (hybridoma and B-cell), in vitro (phage display), and in silico (artificial intelligence and machine learning) technologies to both accelerate and enhance antibody discovery. Individually, each technology is valuable in its own right, but when combined, their collective benefits blend together to create a new gold standard for antibody discovery.
In vivo discovery: hybridoma, single-B cell technology, and humanization
Approximately 500 million years ago, eukaryotic organisms (jawed fish) developed what may be the first adaptive immune systems. The rudimentary proteins that coursed through these ancient animals later gave rise to the complex heterodimeric proteins that we now recognize as immunoglobulins, otherwise known as antibodies1. Today, all jawed vertebrates express a vast and diverse array of antibody variants that help the body recognize and eliminate foreign molecules.
B lymphocytes are one of many important cell types that mediate this process. B cells undergo somatic recombination to generate a large repertoire of unique antibody sequences. And, when a B cell’s antibody recognizes an antigen, it catalyzes a chain reaction that not only prompts an immune response, but also leads to the subsequent clonal expansion of that antibody sequence such that an army of B cells are produced with the same antibody sequence.
in vivo approaches to antibody discovery take advantage of this system, tapping into the vast library of antibodies that are naturally present in a host animal and its ability to amplify antigen-specific sequences. Among the most popular in vivo approaches are those based on hybridoma and single-B cell technologies.
Hybridoma technology
Briefly, hybridoma technologies work by first inoculating a host animal with the target antigen. Once an immune response has been initiated, B cells are isolated from the animal. Without intervention, these primary cells would be too short-lived for discovery efforts. To ensure that these cells can be kept alive for long periods of time, the primary B cells are fused with mouse myeloma cells, rendering them immortal. The resulting hybrid myeloma cells (hybridoma cells) can be clonally expanded and subsequently screened for antigen-binding properties3,4.
There are several advantages to hybridoma technologies. Living organisms have evolved maturation processes that prevent the survival of B cells containing truncated or self-aggregating antibodies. This pre-filtering process means that hybridoma-based discovery campaigns generate highly developable antibody clones. Additionally, after inoculation, the expansion of B cell clones are likely biased for antibodies that have already demonstrated a high affinity for the target antigen3,4.
Hybridoma technology has several limitations as well. The process of fusing primary B cells with myeloma cells is inefficient, leading to the loss of more than 99% of input cells and the potential antibody sequences they carry. Additionally, the animal's immune response may reduce diversity in the hybridoma library. After inoculation, there will be an expansion of antibody diversity to increase its odds of producing antigen binders. But, eventually, the diversity of antibody variants contracts so that the bulk of the antibodies are targeting similar epitopes (binding spots on a target antigen). This narrowing effect is both a benefit and a limitation: the antibodies that come out of this are likely to be high quality, but the effective diversity of the library will be smaller than might be possible with other systems3,4.
Summary of hybridoma benefits
Hybridoma technology makes use of immortalized B cells that are produced when mouse myeloma cells are fused with antibody-producing B-cells. Hybridoma cells are commonly used as an enduring source of antibodies for discovery and development purposes. Benefits of using hybridoma cells include:
- in vivo maturation processes eliminate B cells containing self-aggregating, truncated, or otherwise non-functional antibodies, thus leading to an antibody library enriched in functional antibodies that are likely to be developable
- in vivo affinity maturation enriches B cell populations for higher affinity antigen binders
- Antibodies preselected for natural heavy- and light-chain pairings
Note that humanization will likely be used whenever starting with non-human antibody sources.
Single-B cell screening
Single-B cell technology is an evolution from hybridoma technology, carrying many of the same advantages (namely in vivo affinity maturation and antigen-binding biases) but with a few significant improvements.
Rather than risk losing antibodies during the fusion process, researchers can screen considerably more cells and antibody sequences by circumventing the need for fusion with myeloma cells. Instead, primary B cells are isolated and assayed for their antigen-binding qualities using single-cell analytical methods, like flow cytometry or microfluidic cell manipulation. With Beacon technology, B cell screening can be multiplexed, such that multiple data points can be collected for each cell in order to better describe its antigen-binding properties. Single-cell sequencing of selected b cells may reveal antibody sequences within just a week—obviating the need for prolonged culture5.
Additionally, whereas the hybridoma process may take weeks to complete, single-B cell screening can be done in a day. Unlike hybridoma-based methods, which are largely restricted to the use of rodent cells, B cell screening makes it possible to harvest antibodies from a wide range of species, even humans. Such an approach can be a valuable way to rapidly identify antibodies with therapeutic potential during infectious disease outbreaks, for instance5.
Taken together, single-B cell sequencing technology enables more efficient and large-scale in vivo antibody discovery.
Summary of single-B cell sequencing benefits
B Cell Sequencing is an approach to antibody discovery wherein primary B cells are isolated and assayed for their antigen-binding qualities using single-cell analytical methods. Importantly, B cells can be sourced from myriad species, including humans. Benefits of B cell sequencing include:
- in vivo maturation processes eliminate B cells containing self-aggregating, truncated, or otherwise non-functional antibodies
- Antibodies preselected for natural heavy- and light-chain pairings
- Greater efficiency achieved by circumventing the need for cell fusion, enabling much of the B cell library to be included in the discovery process
- Higher throughput and potential for single day turnaround
- Enables use of human B cell repertoire
- Can be multiplexed to allow for multiple data points gathered from a single B cell
ヒト化
Therapeutic antibodies derived from non-human animals must go through a process known as humanization wherein key elements of the candidate antibody are transported (or grafted) into a human antibody sequence. Briefly, antibodies contain a group of amino acid loops (known as complementarity determining regions, or CDRs) that play a fundamental role in determining which antigen each antibody will recognize. The rest of the antibody is largely conserved within species. Humanization is the process of grafting CDRs from the original antibody into a human antibody sequence, thereby producing an antibody that not only binds to the target antigen of interest, but contains mostly human sequence content6.
Beyond just grafting CDRs, today’s humanization technology enables the generation of humanized antibodies that also closely replicate structural elements of the original antibody that are critical for antigen recognition2. With modern computational modeling and machine learning-based algorithms, researchers can preserve the original antibody’s pharmacological properties while eliminating immunogenic sequence content with precision7. Immunogenicity is a concern for all antibodies (even fully human antibodies), but with the right tools and expertise, humanized antibodies can be no more immunogenic than their fully human counterparts.
🧬 Humanization With a Twist
Twist is a world-leader in humanization, making use of a machine learning-assisted approach to render candidate antibodies in a human framework. And, with high-throughput IgG antibody production capabilities, humanized antibody clones can be synthesized and tested.
In vitro antibody discovery: ファージディスプレイ
In relying on a host animal’s ability to generate antibodies, researchers give up a certain degree of control over the diversity of the resulting library pool. Some researchers may wish to be more precise in library construction, defining the types of antibody variants made and the selection criteria. For that, they turn to in vitro technology.
Advances in DNA sequencing technology have made it possible to synthesize fragments of antibody-binding domains en mass for screening purposes. The DNA coding for these domains can be inserted into a phagemid vector in such a way that the antibody domain is expressed on the surface of a bacteriophage. This is the basis of phage-display technology8.
In short, libraries containing single-chain variable fragments (scFv), variable heavy domain of heavy chain fragments (VHH), or antigen-binding fragments (Fab) can be synthesized on a large scale. Heterologous expression of these fragments on the surface of bacteriophage allows for a process known as biopanning wherein the fragment-laden phage are washed over an antigen-coated surface. Only those phage that bind to the antigen will remain after washing. Sequencing of the bound phage reveals the underlying antibody sequence8,9.
Such an approach brings a new scale, throughput, and control to antibody discovery. Whereas, the diversity of in vivo libraries can flex and contract in response to an antigen, phage-display libraries are static, meaning researchers can precisely define the breadth of antibody diversity in their library with as many as 1011 unique antibody variants9.
A significant benefit of phage-display technology is that antibody fragments can be synthesized within human frameworks, eliminating the need for humanization and the ill effects it may have on antibody properties. Additionally, the ability to screen different antibody fragment types can be advantageous when developing biologics for cell therapies. Lastly, the high degree of control provided by phage-display enables researchers to carefully select for desired antibody properties with more precision than can be achieved by in vivo technologies8,9.
However, as with all technologies, phage display has its limitations. Relative to in vivo technologies, phage display requires considerable trial and error to find optimal pairings of antibody heavy- and light-chains. While it is possible to overcome this limitation, doing so adds scale and additional layers of complication to a process that is already technically challenging.
Twist has developed a collection of antibody libraries that are general, naïve, and target-focused, with each having been pre-screened for liability motifs. As such, Twists’ library of libraries can help make phage-display a more efficient discovery method.
Summary of phage display benefits
Phage display is a commonly used tool in antibody discovery and development wherein bacteriophage are engineered to produce antibody fragments that are tethered to viral surface proteins. Benefits of Phage display include:
- Fixed diversity that is custom designed to match research needs
- Fragments synthesized in human frameworks, obviating the need for humanization
- Customization and rationally designed diversity allows for more precise selection of antibody qualities
- Diversity in epitope recognition can be preserved
- Variety of antibody fragment formats including scFv, VHH, and Fab (important for cell therapy development)
Note that humanization will likely be used whenever starting with non-human antibody sources.
In silico antibody development: artificial intelligence and machine learning
Fundamentally, antibody discovery and development is an exercise in serial decision-making. At every stage, decisions have to be made about which antibodies are worth testing, the features that will define a promising candidate, and where optimization efforts should focus. The quality of these decisions—and the resulting antibody product—depends largely on the quality of the tools that inform them.
In recent years, in silico tools have become increasingly integrated into the decision-making process. To describe the many ways that these technologies are applied in this space is beyond the scope of this article (an in-depth review can be found here). In general, tools such as artificial intelligence (AI) and machine learning models (ML) allow researchers to design and interrogate larger pools of antibody candidates, specifically enabling non-obvious pattern recognition. These tools can be beneficial throughout the discovery and development process, from designing phage display libraries to predicting the developability of candidates10.
AI and ML tools have found particular usefulness in guiding the process of directed evolution, wherein antibody qualities are progressively improved by using the most promising candidates from a screen as the basis of subsequent screening libraries. This process involves designing antibody clones that contain variation at either the amino acid or domain level. In this way, antibodies can evolve with each iterative cycle.
However, traditional directed evolution is limited in several ways. Selecting for multiple antibody traits is challenging, and what benefits one property may detract from another. Additionally, the process can be biased against rare clones, potentially causing the dropout of therapeutically valuable candidates.
In combination with structural modeling and next-generation sequencing, ML and AI tools can help overcome these challenges by recognizing non-obvious links between an antibody’s sequence, structure, and properties. This information can then be used to improve the diversity of antibody candidates while also enriching desired properties—such as antigen binding affinity, target specificity, biological efficacy, hydrophobicity, and self-aggregating potential among many others. These tools can also guide optimization efforts by highlighting key loci where modification of the antibody is likely to be most effective.
In short, a suite of in silico tools is available to help researchers better design and analyze antibody candidates, ultimately improving the quality of antibodies that progress through the pipeline.
Summary of in silico benefits
In recent years, many in silico tools have been developed to support antibody discovery and development, ranging from artificial intelligence and machine learning to structural modeling. Here are a few of the many benefits of these tools:
- Artificial-intelligence-assisted library design ensures that the initial antibody library samples a diversity of the sequencing space without wasting resources on high-liability, low-developability sequences.
- Unsupervised machine learning tools support lead picking by analyzing NGS data from screening campaigns, maximizing sequence diversity.
- Machine Learning methods have been developed to assist with lead optimization, for example, humanization to reduce immunogenicity.
- Machine Learning algorithms have also been trained to identify which candidates are likely to have superior biophysical properties such as reduced hydrophobicity.
Towards a new gold standard
As you can see, there are multiple approaches to antibody discovery that each have advantages and limitations. Historically, researchers have had disparate access to in vivo, in vitro, and in silico tools when beginning the antibody discovery process. Whether they commit to one technology or the other often has more to do with tradition and available resources than scientific reason.
But what if there was a better way, one that brought together the strengths of each approach to maximize antibody discovery potential?
Twist Biopharma Solutions has recently made it easier to do exactly that by assembling a suite of antibody discovery tools under one roof. Twist’s combined offering includes its unparalleled synthesis platform that is used to create phage-display libraries containing billions of variants; the use of the advanced DiversimAb(™) mouse for hybridoma-based screening; and Beacon-based single-B cell sequencing for high-throughput, multiplexed B-cell screening from multiple different species, including mouse, rabbit, and alpaca. Each technology can be used individually. But when combined, they enable researchers to maximize their shots on goal through massively parallel antibody screening.
Importantly, Twist’s antibody discovery platform incorporates ML and AI to aid in both library design and screening. These tools not only help to identify candidate sequences that are most likely to succeed, but they allow promising candidates to be recognized even when they’re under-represented in the screening library.
Individually, each technology in Twist’s platform is valuable, but when combined, the exclusivity of antibody discovery tools dissolves away, leaving researchers with a new gold standard.
参考文献
- Oreste, Umberto, et al. “On Origin and Evolution of the Antibody Molecule.”Biology, vol. 10, no. 2, 10 Feb. 2021, p. 140, pubmed.ncbi.nlm.nih.gov/33578914/, https://doi.org/10.3390/biology10020140.
- Ducancel, Frédéric, and Bruno H. Muller. “Molecular Engineering of Antibodies for Therapeutic and Diagnostic Purposes.”MAbs, vol. 4, no. 4, July 2012, pp. 445–457, www.ncbi.nlm.nih.gov/pmc/articles/PMC3499340/pdf/mabs-4-458.pdf, https://doi.org/10.4161/mabs.20776.
- Moraes, Jane Zveiter, et al. “Hybridoma Technology: Is It Still Useful?”Current Research in Immunology, vol. 2, 2021, pp. 32–40, https://doi.org/10.1016/j.crimmu.2021.03.002.
- Mitra, Sanchita, and Pushpa Chaudhary Tomar. “Hybridoma Technology; Advancements, Clinical Significance, and Future Aspects.”Journal of Genetic Engineering & Biotechnology, vol. 19, no. 1, 18 Oct. 2021, p. 159, www.ncbi.nlm.nih.gov/pmc/articles/PMC8521504/, https://doi.org/10.1186/s43141-021-00264-6.
- Pedrioli, Alessandro, and Annette Oxenius. “Single B Cell Technologies for Monoclonal Antibody Discovery.”Trends in Immunology, vol. 42, no. 12, 1 Dec. 2021, pp. 1143–1158, www.cell.com/trends/immunology/fulltext/S1471-4906(21)00213-1, https://doi.org/10.1016/j.it.2021.10.008.
- Jones, Peter T., et al. “Replacing the Complementarity-Determining Regions in a Human Antibody with Those from a Mouse.”Nature, vol. 321, no. 6069, 5月 1986, pp. 522–525, https://doi.org/10.1038/321522a0.
- Marks, Claire, et al. “Humanization of Antibodies Using a Machine Learning Approach on Large-Scale Repertoire Data.”Bioinformatics, 10 June 2021, https://doi.org/10.1093/bioinformatics/btab434.
- Alfaleh, Mohamed A., et al. “Phage Display Derived Monoclonal Antibodies: From Bench to Bedside.”Frontiers in Immunology, vol. 11, 28 Aug. 2020, https://doi.org/10.3389/fimmu.2020.01986.
- Lu, Ruei-Min, et al. “Development of Therapeutic Antibodies for the Treatment of Diseases.”Journal of Biomedical Science, vol. 27, no. 1, 2 Jan. 2020, jbiomedsci.biomedcentral.com/articles/10.1186/s12929-019-0592-z, https://doi.org/10.1186/s12929-019-0592-z.
- Kim, Jisun, et al. Computational and Artificial Intelligence-Based Methods for Antibody Development. Vol. 44, no. 3, 1 Mar. 2023, pp. 175–189, https://doi.org/10.1016/j.tips.2022.12.005.
研究専用です。Not for use in diagnostic procedures.
いかがでしたか?
気に入った
気に入らなかった
大好き
驚かされた
面白かった